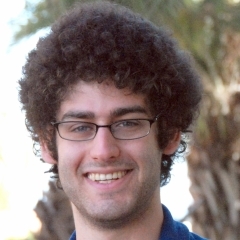
Date:
Location:
Speaker:
Title: Measuring Biochemical Possibility Spaces in Evolutionary Engineering
Advisors: Irene Chen and Siddharth Dey
Abstract:
At the molecular level, artificial selection—improving or designing new biocatalysts and reagents by controlling evolution—is one of the most versatile tools in biochemical engineering. But fully harnessing evolution requires knowledge of the shape and dynamics of complete evolutionary spaces. Prior to this work, very little research has measured the real chemical and evolutionary dynamics of artificial selection. By updating the classical theory of simple selections towards an engineering focus, and combining this with direct observations of direct evolving populations, we show here the first comprehensive descriptions of how whole populations evolve during the selection of novel biocatalysts.
This work seeks to address this at several levels. First, we offer a theoretical approach to mapping the distribution of fitness effects in any system under driven selection. Broadly, this solution makes it possible to approximate the overall distribution of any selectable chemical function across random molecular space. Zooming in, we next develop high-throughput tools to view an entire population of active catalysts as it dynamically changes over the course of an entire selection. Our results show that RNA-based triphosphorylation catalysis is log-normally distributed over polymer sequence space. We also present the first picture of non-ideality during a real selection, demonstrating that stochastic effects can have a powerful and confounding impact on engineered evolution. Finally, we investigate the evolution of an RNA aminoacylase whose emergence may have been crucial to the origin of the genetic code. Using a new workflow we term Sequencing to determine Catalytic Activity Paired with Evolution (SCAPE), we build the first large, dynamic landscape of chemical activity. The resulting data set measures catalytic activity of millions of evolved biomolecules simultaneously, pairing kinetic variations with genetic sequence at single nucleotide resolution, and building the first complete map of all evolutionary pathways to an engineered function from anywhere in genetic space.
Our methods and results suggest general applicability to more complicated systems, with implications for A) measuring the parameters necessary to fully optimize genetic engineering, and B) measuring the evolutionary emergence and distribution of a wide range of biocatalytic functions.