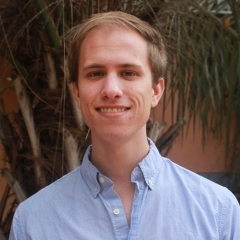
Date:
Location:
Speaker:
Title: “Importance learning: new methods to efficiently model heterogeneous catalysts with quenched disorder”
Abstract: Over the past few decades there have been tremendous advances in the accuracy of electronic structure calculations, rigorous methods to assess proposed reaction mechanisms, and techniques to create representative models of active sites for homogeneous and ordered heterogeneous catalysts. Advances on all these fronts have greatly improved our understanding of numerous catalysts. However, most of these methods assume that the active sites are both well-defined and uniform (or are in an ensemble of uniform sites). Catalysts with quenched disorder have non-uniform active sites. Most established modeling techniques are inapplicable and many important catalysts in this category remain poorly understood. The major challenges of modeling catalysts with quenched disorder are (i) the nature of the disorder is out-of-equilibrium and unknown; (ii) each active site has a different local environment and activity; (iii) active sites are rare, often less than ~20% of potential sites, depending on the catalyst and preparation method.
In this thesis, we develop new methods to efficiently predict kinetic properties of the distribution of quenched-disordered sites and how they affect different steps of catalyst operation. We combine population balance modeling techniques with machine learning methods to learn how the population of grafted sites evolves in time. We develop a new algorithm to efficiently converge site-averaged kinetics for a given distribution of grafted sites, the Importance Learning algorithm. A combination of Importance sampling and machine Learning are used to efficiently and accurately learn activation parameters of rare sites that dominate site-averaged kinetics. Finally, we develop a protocol to create cluster models of active sites that most accurately preserve quenched degrees of freedom.